Unlock the Power of Statistical Learning for Dependent Data: Dive into Statistical Learning and Dependent Data Springer Texts in Statistics

In the realm of data analysis, understanding the complexities of dependent data is paramount. Statistical Learning and Dependent Data Springer Texts in Statistics empowers data scientists, statisticians, and researchers with a comprehensive guide to tackling the challenges posed by correlated observations. This groundbreaking book provides a deep dive into the theory, methods, and applications of statistical learning for dependent data, equipping readers with the skills and knowledge to extract meaningful insights from complex datasets.
Delving into the World of Dependent Data
Statistical dependence, a phenomenon where observations are not independent of each other, introduces unique challenges in data analysis. Traditional statistical methods, designed for independent data, can yield misleading results when applied to dependent data. Statistical Learning and Dependent Data Springer Texts in Statistics addresses this critical issue by introducing specialized techniques tailored specifically for handling dependent data.
5 out of 5
Language | : | English |
File size | : | 10730 KB |
Screen Reader | : | Supported |
Print length | : | 631 pages |
The book begins by laying a solid foundation in the fundamental concepts of statistical dependence, including measures of association, correlation structures, and time series analysis. It then delves into a wide range of statistical learning methods adapted for dependent data, such as:
- Generalized Linear Models (GLMs): Extended for dependent data, GLMs incorporate correlation structures to account for the non-independence of observations.
- Generalized Additive Models (GAMs): These non-parametric models offer flexibility in capturing complex relationships between dependent variables and multiple independent variables.
- Kernel Methods: Leveraging powerful kernel functions, these methods can uncover non-linear dependencies and identify patterns in high-dimensional data.
- Bayesian Methods: Bayesian approaches provide a probabilistic framework for modeling dependent data, allowing for uncertainty quantification and incorporation of prior knowledge.
Applications in Various Domains
The applicability of statistical learning for dependent data extends across a vast array of fields, including:
- Biostatistics: Analyzing longitudinal data, modeling clustered outcomes, and identifying risk factors in complex healthcare settings.
- Finance: Forecasting financial time series, assessing risk, and optimizing portfolio allocation considering dependencies among assets.
- Marketing: Understanding customer behavior, segmenting markets, and predicting consumer preferences in the presence of dependent observations.
- Environmental Science: Modeling spatiotemporal data, analyzing climate patterns, and assessing environmental impacts with consideration of spatial and temporal correlations.
Key Features and Benefits
Statistical Learning and Dependent Data Springer Texts in Statistics offers numerous advantages:
- Comprehensive Coverage: Provides a comprehensive overview of statistical learning for dependent data, covering both theory and applications.
- Rigorous Mathematical Foundation: Grounded in robust mathematical principles, ensuring the validity and reliability of the methods presented.
- Practical Examples and Case Studies: Includes real-world case studies and hands-on examples illustrating the practical implementation of the techniques.
- Accessible Language: Written in a clear and accessible style, making it suitable for readers with a wide range of backgrounds.
- Extensive References: Provides an exhaustive list of references for further exploration and in-depth research.
Who Should Read This Book?
Statistical Learning and Dependent Data Springer Texts in Statistics is an invaluable resource for:
- Data scientists seeking advanced techniques for handling dependent data.
- Statisticians interested in expanding their knowledge in the field of dependent data analysis.
- Researchers looking to tackle complex data challenges involving correlated observations.
- Graduate students pursuing degrees in statistics, data science, or related fields.
Statistical Learning and Dependent Data Springer Texts in Statistics is an indispensable guide to the world of dependent data analysis. By providing a comprehensive overview of statistical learning methods tailored for dependent data, this book empowers readers to unlock the full potential of their complex datasets. Whether you are a data scientist, statistician, or researcher, this book will equip you with the knowledge and skills to extract meaningful insights, improve decision-making, and advance your research in a wide range of domains.
5 out of 5
Language | : | English |
File size | : | 10730 KB |
Screen Reader | : | Supported |
Print length | : | 631 pages |
Do you want to contribute by writing guest posts on this blog?
Please contact us and send us a resume of previous articles that you have written.
Book
Novel
Page
Chapter
Text
Story
Genre
Reader
Library
Paperback
E-book
Magazine
Newspaper
Paragraph
Sentence
Bookmark
Shelf
Glossary
Bibliography
Foreword
Preface
Synopsis
Annotation
Footnote
Manuscript
Scroll
Codex
Tome
Bestseller
Classics
Library card
Narrative
Biography
Autobiography
Memoir
Reference
Encyclopedia
Svetlin Georgiev
Steve Swayne
Shannon Dittemore
Sonia Levitin
Vincent Helices
Shyla Esko Bare
Shekhar Guha
Shannon Lee Alexander
Robert Nichols
Robert Grant
Maurene Goo
Mike Critchley
Serene Conneeley
Rudolf Grafe
Shikha Sachdeva
Russell Sullivan
Rl Stoll
Shannon Rizzotto
Rowland Hayler
Stavros Karathanasis
Light bulbAdvertise smarter! Our strategic ad space ensures maximum exposure. Reserve your spot today!
- Ervin BellFollow ·11.2k
- Herman MelvilleFollow ·5.3k
- Brandon CoxFollow ·14.6k
- Noah BlairFollow ·3.9k
- Harrison BlairFollow ·11k
- José SaramagoFollow ·15.1k
- Forrest BlairFollow ·18.3k
- J.D. SalingerFollow ·5.4k
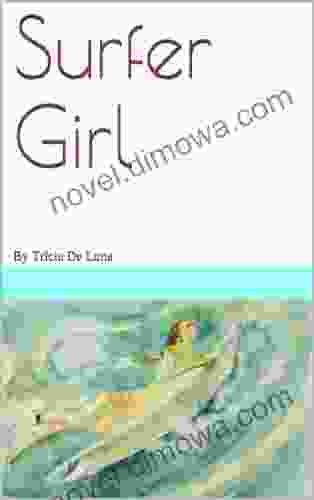

Ride the Waves with "Surfer Girl" by Tricia De Luna: A...
Prepare to be...
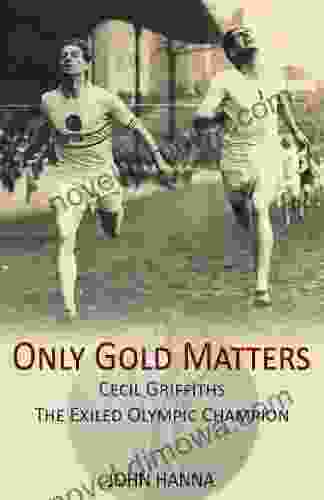

Cecil Griffiths: The Exiled Olympic Champion
Cecil Griffiths was...
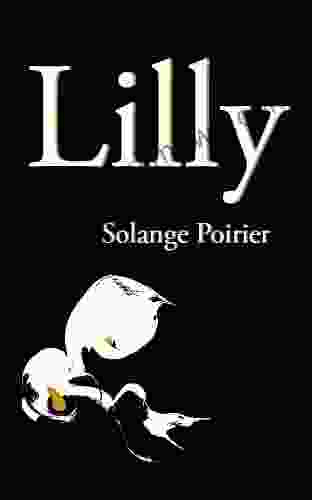

Lilly Blue Iris Stanley Gene: A Captivating Literary...
Enter a Realm of...
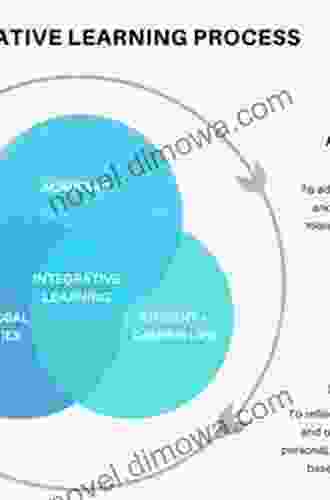

From Vision to Implementation: An Integrative Approach to...
Unleash the Power...
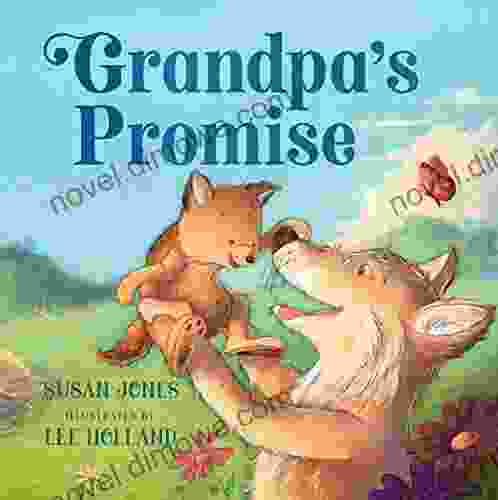

Discover the Heartwarming Journey of a Grandfather and...
In a quaint little town nestled amidst...
5 out of 5
Language | : | English |
File size | : | 10730 KB |
Screen Reader | : | Supported |
Print length | : | 631 pages |