Step By Step Classifying Images With Python And Techniques Of Computer Vision

Image classification is a fundamental task in computer vision. It involves assigning a label to an image, based on its content. Image classification has a wide range of applications, such as object detection, scene recognition, and medical diagnosis.
5 out of 5
Language | : | English |
File size | : | 6286 KB |
Screen Reader | : | Supported |
Print length | : | 254 pages |
Lending | : | Enabled |
Paperback | : | 308 pages |
Reading age | : | 10 - 12 years |
Grade level | : | 5 - 6 |
Item Weight | : | 1.17 pounds |
Dimensions | : | 6 x 0.7 x 9 inches |
Hardcover | : | 120 pages |
In this tutorial, we will learn how to classify images using Python and techniques of computer vision. We will cover the following topics:
* Loading and preprocessing images * Extracting features from images * Training a classifier * Evaluating the performance of a classifier
Loading and Preprocessing Images
The first step in image classification is to load and preprocess the images. This involves resizing the images to a consistent size, converting them to a common format, and normalizing the pixel values.
Here is an example of how to load and preprocess an image using Python:
import cv2
# Load the image image = cv2.imread("image.jpg")
# Resize the image image = cv2.resize(image, (224, 224))
# Convert the image to RGB format image = cv2.cvtColor(image, cv2.COLOR_BGR2RGB)
# Normalize the pixel values image = image / 255.0
Extracting Features from Images
Once the images have been loaded and preprocessed, the next step is to extract features from them. Features are numerical representations of the image that can be used to train a classifier.
There are many different ways to extract features from images. One common approach is to use a convolutional neural network (CNN). CNNs are a type of deep learning model that is specifically designed for image processing.
Here is an example of how to use a CNN to extract features from an image:
import tensorflow as tf
# Create a CNN model model = tf.keras.models.Sequential([ tf.keras.layers.Conv2D(32, (3, 3),activation="relu", input_shape=(224, 224, 3)),tf.keras.layers.MaxPooling2D((2, 2)),tf.keras.layers.Conv2D(64, (3, 3),activation="relu"),tf.keras.layers.MaxPooling2D((2, 2)),tf.keras.layers.Flatten(),tf.keras.layers.Dense(128, activation="relu"),tf.keras.layers.Dense(10, activation="softmax") ])
# Extract features from the image features = model.predict(image)
Training a Classifier
Once the features have been extracted from the images, the next step is to train a classifier. A classifier is a model that can assign a label to an image, based on its features.
There are many different types of classifiers that can be used for image classification. One common approach is to use a support vector machine (SVM). SVMs are a type of machine learning model that is specifically designed for classification tasks.
Here is an example of how to train an SVM classifier for image classification:
import sklearn.svm
# Create an SVM classifier classifier = sklearn.svm.SVC()
# Train the classifier classifier.fit(features, labels)
Evaluating the Performance of a Classifier
Once the classifier has been trained, the next step is to evaluate its performance. This involves testing the classifier on a new set of images that it has not seen before.
The performance of a classifier is typically evaluated using the following metrics:
* Accuracy: The accuracy of a classifier is the percentage of images that it correctly classifies. * Precision: The precision of a classifier is the percentage of images that it correctly classifies as positive, out of all the images that it classifies as positive. * Recall: The recall of a classifier is the percentage of images that it correctly classifies as positive, out of all the images that are actually positive.
Here is an example of how to evaluate the performance of a classifier:
import sklearn.metrics
# Test the classifier on a new set of images predictions = classifier.predict(test_features)
# Calculate the accuracy, precision, and recall of the classifier accuracy = sklearn.metrics.accuracy_score(test_labels, predictions) precision = sklearn.metrics.precision_score(test_labels, predictions) recall = sklearn.metrics.recall_score(test_labels, predictions)
# Print the accuracy, precision, and recall of the classifier print("Accuracy:", accuracy) print("Precision:", precision) print("Recall:", recall)
In this tutorial, we have learned how to classify images using Python and techniques of computer vision. We covered the following topics:
* Loading and preprocessing images * Extracting features from images * Training a classifier * Evaluating the performance of a classifier
I hope this tutorial has been helpful. If you have any questions, please feel free to leave a comment below.
5 out of 5
Language | : | English |
File size | : | 6286 KB |
Screen Reader | : | Supported |
Print length | : | 254 pages |
Lending | : | Enabled |
Paperback | : | 308 pages |
Reading age | : | 10 - 12 years |
Grade level | : | 5 - 6 |
Item Weight | : | 1.17 pounds |
Dimensions | : | 6 x 0.7 x 9 inches |
Hardcover | : | 120 pages |
Do you want to contribute by writing guest posts on this blog?
Please contact us and send us a resume of previous articles that you have written.
Book
Novel
Page
Chapter
Text
Story
Genre
Reader
Library
Paperback
E-book
Magazine
Newspaper
Paragraph
Sentence
Bookmark
Shelf
Glossary
Bibliography
Foreword
Preface
Synopsis
Annotation
Footnote
Manuscript
Scroll
Codex
Tome
Bestseller
Classics
Library card
Narrative
Biography
Autobiography
Memoir
Reference
Encyclopedia
Ruthie Godfrey
Sally Hull
Ted Macauley
Sigmund Brouwer
Simon Curtis
Terry Frei
Sheldon Axler
Sam Day
Robert J Meurn
Robert Garner
K M Robinson
Amy Hest
Ron Houchin
Tracy Partridge Johnson
Roxy Eloise
Victoria London
Roger Naylor
Sara B Larson
Sarah Withrow King
Shekhar Guha
Light bulbAdvertise smarter! Our strategic ad space ensures maximum exposure. Reserve your spot today!


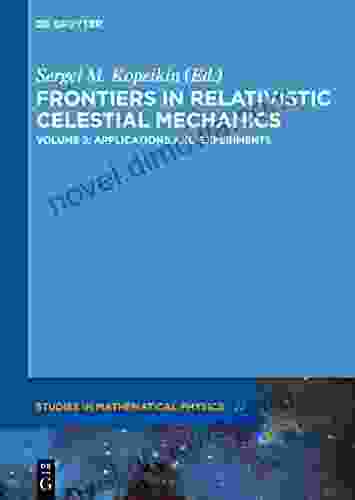

- Carlos DrummondFollow ·5.1k
- Julio Ramón RibeyroFollow ·16.8k
- Norman ButlerFollow ·15.1k
- Stephen FosterFollow ·12k
- Cormac McCarthyFollow ·9.2k
- Giovanni MitchellFollow ·15.3k
- Angelo WardFollow ·18.1k
- Anton FosterFollow ·2.2k
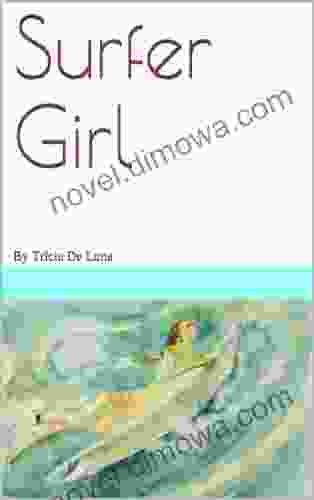

Ride the Waves with "Surfer Girl" by Tricia De Luna: A...
Prepare to be...
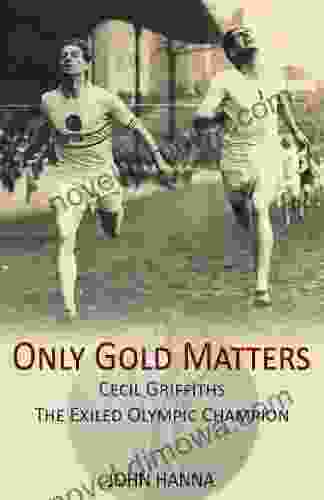

Cecil Griffiths: The Exiled Olympic Champion
Cecil Griffiths was...
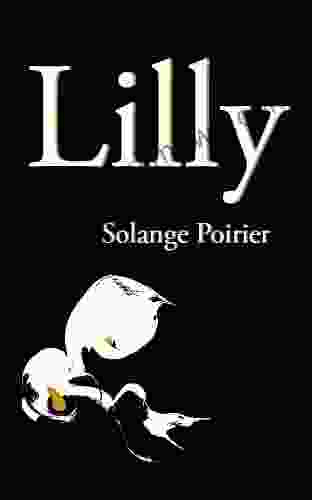

Lilly Blue Iris Stanley Gene: A Captivating Literary...
Enter a Realm of...
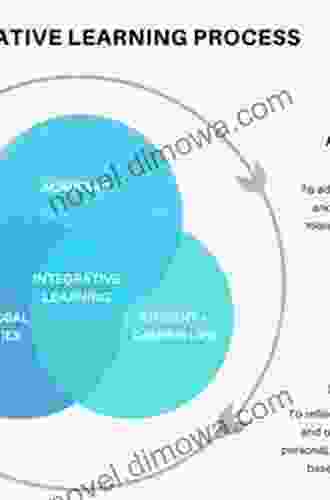

From Vision to Implementation: An Integrative Approach to...
Unleash the Power...
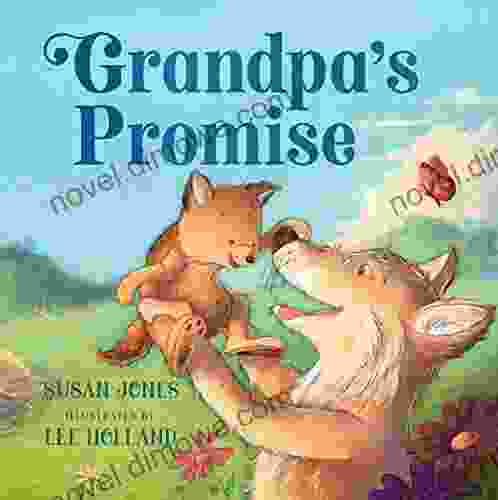

Discover the Heartwarming Journey of a Grandfather and...
In a quaint little town nestled amidst...
5 out of 5
Language | : | English |
File size | : | 6286 KB |
Screen Reader | : | Supported |
Print length | : | 254 pages |
Lending | : | Enabled |
Paperback | : | 308 pages |
Reading age | : | 10 - 12 years |
Grade level | : | 5 - 6 |
Item Weight | : | 1.17 pounds |
Dimensions | : | 6 x 0.7 x 9 inches |
Hardcover | : | 120 pages |